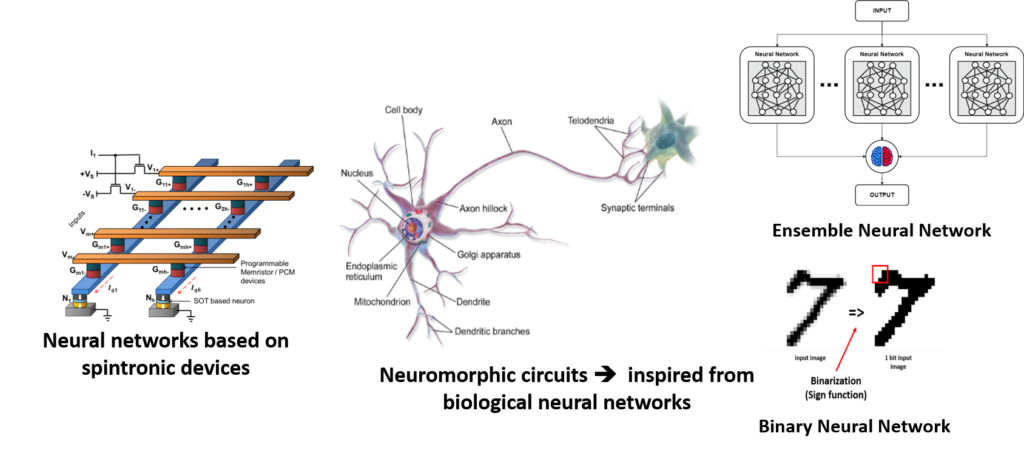
We have investigated the use of spintronic devices to enable the implementation of a Binary Ensemble Convolutional Neural Network. A simulation flow has been setup and allowed to evaluate this disruptive combination and very promising results have been obtained in terms of hardware optimization and power reduction.
The use of emerging technologies has been widely studied to efficiently implement Convolutional Neural Networks (CNN). Indeed, the standard Von-Neumann architecture does not give satisfactory results due to the memory access wall during inference. Crossbar array based on non-volatile resistive devices (memristors) allows performing In-Memory Computing (IMC), help mitigate this issue. Thanks to the resistive nature of devices, basic MAC operations required for CNN is done in an analog manner, inside the data storage block.
However, the use of memristive devices suffers from limitations. The lack of maturity of these emerging technologies results in large process variations affecting the accuracy of the analog computation. Moreover, the binary nature of spintronic devices makes the implementation of multi levels synapses challenging. To mitigate these issues, we propose an architecture which combines two concepts. First, we use Ensemble Neural Network (ENN) paradigm tha relies on the “wisdom of crowds” concept: we replace a large network with smaller and less accurate networks, trained with different samples of the same dataset. We prove that this is particularly well adapted to emerging technologies with possible large process variations. Second, we use binary CNNs that can still provide a very fair accuracy, with an important simplification of the hardware.
The two combined concepts yield a Binary Ensemble CNN (BECNN) based on spintronic memristors. Here the Spin Orbit Torque Magnetic Tunnel Junctions (SOT MTJs) have been used as they offer much higher levels of resistance compared to other technologies. The evaluation of the architecture was performed on several datasets for image recognition. BECNN allows a reduction by 92% of the number of neurons and 95% for synapses respectively, for a similar accuracy. The architecture was implemented on several supports: FPGA, implementation showing already a reduction by 95% and 97% of the number of clock cycles and memory access. Further to that, IMC architecture with SOT crossbars allows a further reduction of the memory access by 95%. The SOT based crossbar array has been designed and validated at circuit level allowing a MAC operation with 3 orders of magnitude lower cost.
Team: Spintronic IC Design
Funding: MIAI @ Grenoble Alpes, (ANR-19-P3IA-0003)
Further reading: Spintronic Memristor based Binarized Ensemble Convolutional Neural Network Architectures, G. T. Tchendjou, K. Danouchi, G. Prenat and L. Anghel, in IEEE Transactions on Computer-Aided Design of Integrated Circuits and Systems, 2022, doi: 10.1109/TCAD.2022.3213612, HAL
Contacts at SPINTEC: Lorena Anghel, Guillaume Prenat