Overview
The transversal team aims at bringing together all competencies from SPINTEC involving spintronic devices nanofabrication, characterization, circuit integration, architecture, and algorithm techniques to implement hardware solutions for artificial intelligence (AI) and unconventional computing.
Spintronic-based multifunctional devices are a substantial opportunity to improve the energy efficiency of next-generation computing hardware. Moreover, this approach allows taking advantage of brain-inspired computing models to deploy cutting-edge neuromorphic algorithms, crossing the gap between current hardware AI implementations and exceptional brain computing ability.
Research topics
Bio-inspired computing
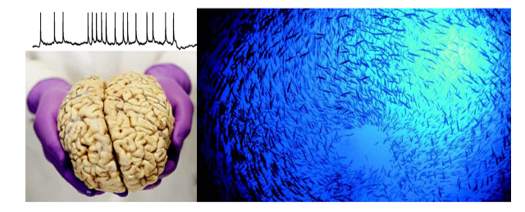
As the brain performs very sophisticated operations and consumes only a few Watts, brain-inspired/neuromorphic computing is a promising path for which spintronic devices can efficiently emulate both neurons and synapses in hardware. Their nanometric size, sensitivity to input stimuli, and interactions make those devices ideal for implementing large arrays of neuro-synaptic elements: spintronic nano-oscillators, spintronic and ferroelectric memristors, magnetic memories, superparamagnetic tunnel junctions, skyrmions, etc.
Probabilistic computing
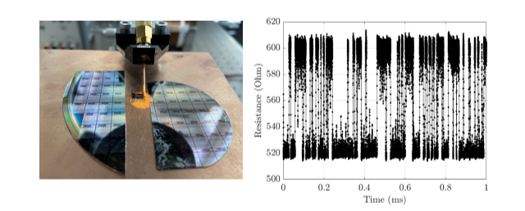
Noise is a crucial ingredient in emulating the stochastic nature of the neural activity and executing energy-efficient computing algorithms such as energy-based or temporal-based machine learning models. In this context, probabilistic computing is a very suitable approach that relaxes usual precision computing constraints. The truly random nature of spintronic devices (such as superparamagnetic tunnel junctions) makes them attractive for hardware implementations of probabilistic computing approaches.
In memory computing
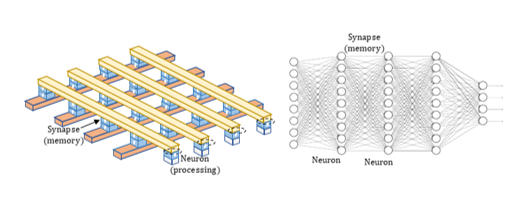
The most promising solutions for non-Von Neumann, in-memory computing architectures are based on the use of emerging technologies, that are able to act as both storage and information processing units thanks to their specific physical properties. High accuracy, Deep neural networks (DNN) can be built with crossbars analog in memory computing concept, involving MRAM families, such as STT, SOT, VCMA, but also with more exotic families of magneto-resistive, and ferroelectric or skyrmion based devices.
The team
Projects
Partners
- CEA LIST
- UMPHY
- CEA LETI
Recent news
- Recruiting a post-doctoral researcher on building spintronic neural networks for pattern recognition (March 29th, 2024)
Context: In the frame of the CEA (PTC-ID) project SpinTrain, Spintec laboratory is opening a postdoctoral researcher position. The candidate will work on stochastic magnetic tunnel junctions grown and fabricated at Spintec, and will use ... - Postdoc offer – Emerging Memory Technologies Testing by Using Machine Learning (March 11th, 2024)
Context: The last decade has seen the emergence of numerous studies around integrated nanoelectronic technologies for a growing number of application areas, ranging from the Internet of Things, to integrated components specialized in the management ... - PhD and Postdoc offer – Exploring novel hardware approaches based on spintronics nano-oscillators for unconventional computing (February 26th, 2024)
One PhD position and one 12 months postdoc position are available for the following topic. Spintronics concepts and devices, such as magnetic tunnel junctions, offer novel low power hardware approaches for implementing unconventional computing schemes. Some ... - seminar – Neuromorphic weighted sum with magnetic skyrmions (February 20th, 2024)
On Wednesday March 13th 2024, we have the pleasure to welcome in SPINTEC Tristan da Câmara presently at Université Catholique de Louvain la Neuve. He will give us a seminar on his work done in ... - Portrait – Louis Farcis, doctorant à SPINTEC (February 13th, 2024)
Louis Farcis est chercheur doctoral à SPINTEC. Son travail concerne les architectures non-conventionnelles pour réaliser des calculs à base de réseaux de neurones spintroniques. Son portrait a été réalisé par l’Institut de Recherche Interdisciplinaire de ...